ICARUS Drone Net
PI Samuel Siewert
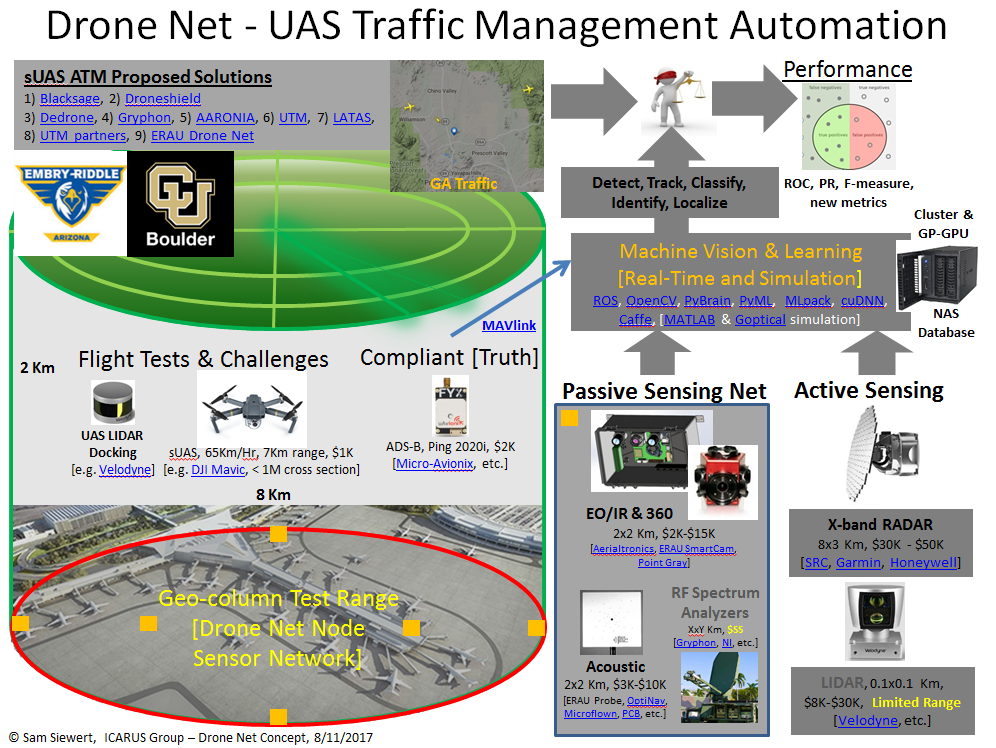
Drone Net is a conceptual architecture to integrate passive sensor nodes in a local sensor network along with traditional active sensing methods for small Unmanned Aerial Systems traffic management. The goal of the proposed research architecture is to evaluate use of multiple passive sensor nodes integrating Electro-Optical/Infrared and acoustic arrays networked around a UAS Traffic Management operating region (Class G uncontrolled airspace). The Drone Net approach will be compared to and/or used in addition to RADAR and Automatic Dependent Surveillance-Broadcast tracking and identification. We hypothesize that this approach can better manage non-compliant small UAS along with compliant UAS and general aviation in sensitive airspace, urban locations, and geo-fenced regions.
Drone-Net: Information Fusion Networks for Reliable Localization
ICARUS Research Group: ERAU Prescott
The challenge and opportunity presented by use of UAS “drones” in the national airspace (NAS) has historic significance not seen since the early days of aviation growth after the First World War. The FAA estimates that by 2020 the drone market will be $98 billion with 7 million drones added annually [1]. Market beneficiaries include industrial inspection, aerial photography, insurance, agricultural and government services [1]. While ADS-B for drones, along with registration, has been proposed as a quick fix, to allow drones into the NAS and to share populated areas, it is not clear how this will work for all types of drones ranging from professional service to hobby. For example, many drones will be fully compliant, but some may be semi-compliant (e.g., low quality position reporting due to degraded GPS), and others perhaps even totally non-compliant or hostile [2].
Embryonic research at ERAU Prescott to develop a drone detector, which can be placed on roof-tops and networked with other detectors and information services, has shown that multi-spectral EO/IR detection is quite effective. The feasibility of passive methods for civil aviation detection of aircraft not using ADS-B and not registered on flilghtradar24 [3] (via primary and secondary RADAR) has been experimentally observed.
The ICARUS group at ERAU Prescott is working to fly and test ADS-B on drones [9] that are compliant, semi-compliant, and non-compliant in order to evaluate methods of detection, classification and identification. Research by Sandia National Labs has shown that drones typically have very low RADAR cross-section area, similar to stealth aircraft [4] and can present a significant security and safety threat. The risk is that even one or two national security incidents involving service drones, hobby, or terrorism could result in the grounding of all drones in the NAS.
Multiple studies [4, 5] have substantiated the conclusion that no single sensing modality will suffice to reliably detect and localize a wide variety of drones. To this end, the ICARUS group proposes to pursue a heterogeneous information fusion approach with passive EO/IR and progressing to a “richer” passive/active sensor suite. Prior ICARUS research partially funded by DHS ADAC Center of Excellence (CoE) led to development of the SMDSI (Software Defined Multi-Spectral Imager) to detect and track marine traffic [6, 7]. This existing hardware will be adapted to accommodate additional sensors including acoustic, ADS-B, primary/secondary RADAR, and LIDAR in order to accelerate the development of optimal methods of drone detection, classification, and identification.
The first year of this proposed effort, will involve basic research in machine learning, machine vision, real-time systems, and the development of suitable information fusion algorithms, continuing through the duration. The test configuration will be documented to facilitate replication at other participating academic research organizations (ERAU Daytona ASSURE [11], U. of Alaska ACUASI [12], U. of Alaska DHS ADAC, and U. of Colorado Boulder Embedded Systems Engineering) in year two of the proposed research. Each collaborator can provide unique synergy to enhance research objectives and are potential future external funding partners. The team will include ERAU Prescott faculty, undergraduate students, CU Boulder graduate ESE students, and collaboration with faculty at U. of Alaska and Colorado. In the third year of work, passive capabilities can be enhanced with active LIDAR and existing RADAR at ERAU Prescott. The goal is to develop unique sensor fusion algorithms as well as machine learning and traditional salient object detection and classification methods.
The images collected over the lifetime of the project can further be saved in a distributed database of observed compliant, compliant-low-quality, non-compliant and perhaps hostile drones and shared between “Drone net” nodes. The overall vision is to create a network of passive/active drone detection, classification, and identification nodes to enhance security and safety for drone operations that surpasses ADS-B and registration alone [10]. The capability can be used to test and evaluate commercial drone detection systems being evaluated by the FBI at airports [8] and to make policy and technology recommendations to key agencies (FAA, DOT, DHS) for drone integration into the national air space. Drone net will enable ICARUS to pursue external funding from DHS CoEs, ONR (Office of Naval Research), NASA AIST (Advanced Information Systems Technology), FAA ASSURE, SBIR/STTR, and industry.
References
[1] FAA Aerospace Forecast, Fiscal Years 2016-2036, Federal Aviation Administration.
[2] How consumer drones wind up in the hands of ISIS fighters, Techcrunch, October 13, 2016.
[3] flightradar24.com, ADS-B, primary/secondary RADAR flight localization and aggregation services.
[4] Birch, Gabriel Carisle, John Clark Griffin, and Matthew Kelly Erdman. UAS Detection Classification and Neutralization: Market Survey 2015. No. SAND2015-6365. Sandia National Laboratories (SNL-NM), Albuquerque, NM, 2015.
[5] McNeal, Gregory S. "Unmanned Aerial System Threats: Exploring Security Implications and Mitigation Technologies." Available at SSRN (2015).
[6] Arctic Domain Awareness Research Center, U. of Alaska Anchorage, Theme 3, Task 2, SmartCam.
[7] S. Siewert, M. Vis, R. Claus, R. Krishnamurthy, S. B. Singh, A. K. Singh, S. Gunasekaran, “Image and Information Fusion Experiments with a Software-Defined Multi-Spectral Imaging System for Aviation and Marine Sensor Networks”, (in preparation) AIAA SciTech, Grapevine, Texas, January 2017.
[8] FAA Tests FBI Drone Detection System at JFK, Federal Aviation Administration, July, 2016.
[9] Micro-Avionix, Ping2020 ADS-B transponder for UAS.
[10] Rhode & Schwarz, Protecting the Sky - Signal Monitoring of Radio Controlled Civilian Unmanned Aerial Vehicles and Possible Countermeasures, October 2015.
[11] ACUASI, Alaska Center for Unmanned Aircraft Systems Integration, http://acuasi.alaska.edu/, Dr. Mike Hatfield.
[12] ASSURE, Alliance of System Safety for UAS through Research Excellence, http://assureuas.erau.edu/, Dr. Richard Stansbury.
Research Dates
04/01/2017 to 03/31/2019
Researchers
Categories: Faculty-Staff
Contact Us
Prescott, AZ 86301